There’s an expression: “Everybody talks about the weather, but nobody does anything about it.” New technology may not be making any inroads in controlling the weather. However, artificial intelligence is helping a select few understand it better and make money from the knowledge.
Venture capital firms are partnering with software engineers to train AI-driven machine learning systems (AIML) to provide a competitive advantage in key industry sectors. The most promising of these is weather prediction. Moreover, hedge funds are seizing on these systems to identify weather-related risks to their portfolios better—be it predicting regional citrus harvests or managing energy generation sources for the electric grid. And achieving advantages over their competitors could be worth tens of billions of dollars.
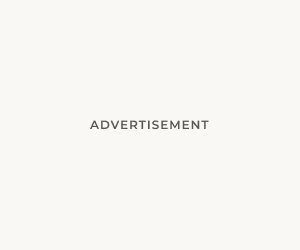
Julie Pullen is a partner at Propeller Ventures, a VC firm specializing in businesses developing ocean and climate technologies. She is building teams to use AIML techniques to enable organizations to customize forecasts for their commercial or scientific needs. Such systems can use proprietary and unique data sources for focused and precise short- and long-term weather prediction not currently available from government sources.
A research scientist at Columbia University’s Earth Institute and former head of the U.S. National Maritime Security Center, Pullen has stepped into the venture capital world to apply her meteorology, oceanography, and climate science knowledge to train AIML systems.
“I see a lot of companies being formed around teams of data scientists to improve weather prediction with AI,” Pullen says, citing Google GraphCast, Huawei Pangu, and Nvidia FourCastNet. “There is a lot of competition entering the field. My team is working on achieving a deeper understanding of the Earth’s atmosphere and oceans for people across an array of disciplines and industries.”
Hedge funds are not only eager customers of weather information; their relative success in using it to improve their portfolios (or not) is an excellent way to validate AIML algorithms.
Customizable Forecasts for Investors
Until recently, weather prediction was such a high-end computing problem that only government agencies, such as the U.S. National Weather Service or the European Center for Medium-Range Weather Forecasts, could pull it off. Moreover, weather forecasting requires many sensors—satellites, ground stations, aircraft, ocean buoys, and other sources—to inform the models.
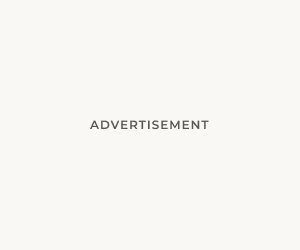
While forecasting has made great strides in recent decades, the scarcity of government service providers, reliable as they are, means everybody essentially has access to the same data. This is great for people planning their day, but it does not offer a competitive advantage to firms whose fortunes depend on atmospheric conditions.
Weather is a valuable industry because everybody needs information about it at some level. With the primary data sources homogenized, the opportunity is to customize forecasts for specific users who then apply them to their activities.
For example, IBM acquired its Weather Company business in 2015, integrating the company’s assets with its own data analysis, presentation, and Watson AI tools. In August, IBM signed a deal to sell The Weather Company to private equity firm Francisco Partners. However, IBM says it will continue providing software support and development. But acquisitions or partnerships like these have their limits: The sources of raw data are essentially the same as what everybody else gets—largely from government agencies.
Satellites, radar, terrestrial stations, and oceanographic sensors feed data into the great, government-supported forecasting service computing systems, which analyze them using some of the most complicated software systems on the planet. The atmosphere is a tremendous fluid, and few things are harder to model than a fluid. One of the areas for improvement of government weather service-supplied information is that the massive computing power needed to process complex models limits the number of forecasts to a few per day.
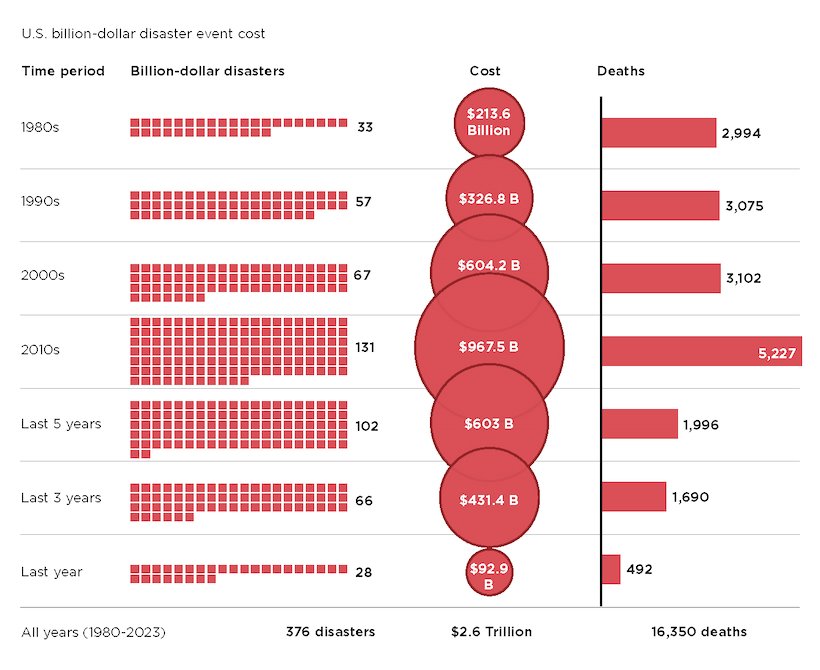
Trained AIML systems can make calculations based on pattern recognition processes much more quickly and as accurately, according to a study published in the journal Science. Another advantage is AIML’s ability to take in various unstructured and custom datasets, including text documents, photographs, audio, video, and other sources not in a machine-recognized format. The key is in the training: Specialists teach the AI to recognize patterns and readings from historic data sources, formatting unstructured data as needed. These may come from government weather services, scientific research, and even fossils and paleoceanography (e.g., core samples of arctic ice or deep ocean mud) from millions of years ago.
Expanding Risk Assessment with AI
Julie Pullen says the widespread affordability of AIML platforms means that individual systems can be trainable in a wide range of specific tasks and open to an expanding range of sources to inform their analyses.
“Venture capital is actively making bets in that space,” Pullen says. “And one of the best ways of getting feedback on those bets is from the hedge fund industry when it uses those tools. It’s the intersection of the scientific with the financial that demonstrates value for predictions along a range of timescales.”
“Hedge funds are seizing on these AI systems to identify weather-related risks to their portfolios better—be it predicting regional citrus harvests or managing energy generation sources for the electric grid.”
She points out that the trainable, customizable features of AIML enable hedge fund managers to look at very specific weather conditions with fine regional detail. Funds can better predict extreme weather events such as heat waves and droughts on time horizons ranging from weeks to months to years. These forecasts make a difference to the bets fund managers make. Then they can get rapid feedback on the models, looking at results from shorter time horizons.
According to Harun Dogo, manager of quantitative analysis at Los Angeles-based hedge fund TCW, widely used weather models are informative. But they make positions in certain commodities expensive to hold because everybody comes to the same conclusion. These widely held positions also come with various levels of risk, not just because they are expensive but also because, at some point, unexpected events—weather-related or geopolitical—will intervene to undermine the common wisdom and destabilize markets. The trick is to be able to make bets against the prevailing wisdom in time and be right about them.
“Artificial intelligence, and really it’s machine learning, is a way to classify patterns in the market and use that information to optimize your positioning,” Dogo says. “What do you think the expected volatility will be? A machine learning algorithm can help you identify risks you didn’t even know you had, things that other quantitative analysts might have missed.”
For example, the world has transitioned from a La Niña weather cycle over the Pacific to an El Niño cycle that shifts global rainfall patterns. Funds dealing in soft commodities (e.g., cocoa futures), energy, and even mining will factor how these predicted patterns may affect portfolios incorporating these sectors. However, everybody has this information. Dogo says AILM offers the opportunity to look at very specific localities on shorter time horizons and discern patterns not covered by historic models of La Niña-El Niño periods.
“So really, the question is, what’s the new information that AIML-enabled climate or weather datasets add to your ability to think about where a particular asset pricing will go?” Dogo says. “How volatile [is it] going to be and what the potential downsides of holding it on particular time horizons will be.”
“Funds can better predict extreme weather events such as heat waves and droughts on time horizons ranging from weeks to months to years. These difference to the bets fund managers make.”
TCW works with third-party partners to receive AIML information to supplement its analysis activities. At this state of the technology’s development, it is not clear that having an in-house capability is worth the time or the resources. In January, Nvidia announced an agreement with datacenter operator Equinox to offer corporate clients AI and machine learning systems. The field is still in its infancy, and questions of whether AIML is better as a third-party service, or an in-house proprietary tool have yet to be answered.
Hedge fund Citadel reportedly made a significant investment in scientists and computer engineers to develop in-house forecasting tools for giving it competitive advantage for trading commodities, especially in the energy sector. Last year, the Financial Times reported that the weather team helped the company earn $16 billion in 2022 to become the most successful hedge fund. The team produces forecasts that are focused on conditions in regions where opportunities for trading in raw materials are most promising.
Hedge Funds Mirror Scientific Researchers
The advent of AIML systems that can run on modest networks of desktop computers is expanding the scope of private weather prediction, opening a new professional track for meteorologists. The website eFinancialCareers.com says meteorologist is becoming a hot job opportunity at hedge funds, with top annual salaries north of $1 million.
From Pullen’s perspective, hedge funds are great as enablers of AIML systems development and validators of analyses and forecasts from such systems. Her team at Propeller is not specifically interested in hedge funds as clients: Its mission is nurturing technologies for improving ocean and climate conditions. However, Pullen is focused on the incredible potential of AILM systems for better understanding marine and weather dynamics on Earth. It just so happens that hedge funds value this information as well and offer an excellent proving ground for the technology.
While hedge funds are notoriously secretive organizations and are not prone to sharing their competitive advantages openly, their requirements for using unstructured data from a variety of sources mirror those of the scientific community. The convergence of science with better financial returns is a powerful dynamic for proving AIML algorithms.
Gordon Gould at San Francisco-based hedge fund Numerai is developing an AIML model that takes information supplied by data scientists to predict the stock market. The fund pays out to subscribers based on how good their data is at enabling the algorithm to foresee market movements. The providers whose data make the most money get the best returns. This sort of on-the-fly data validation is invaluable for better training AIML systems.
AI Opens Up New Business Understanding
The ability to incorporate and analyze unstructured data will be what separates AIML systems from each other. That’s where the competitive advantage lies. It is also how we will better understand our rapidly changing world.
The reliable, routine sources of data that make it into the government service forecasts and supply private weather services are not going to be enough for hedge funds and other users hungry for information from multiple sources in granular detail. Nor, Julie Pullen adds, will it be enough for the development of breakthrough AILM systems for better understanding the Earth and its atmosphere and oceans.
“It’s the datasets that don’t get incorporated into the top forecasts that you want,” she says.
Mainstream forecasts are not necessarily getting data sets acquired by, for example, scientists who might be going out on a research cruise, dropping sensors over the side of a ship, or releasing weather balloons off the deck. There are a lot of observations going on by commercial ships and aircraft just going about their routine business that could be valuable. Private companies are getting into the instrumentation business, including satellites.
Pullen says that part of the challenge in building standout AILM systems is identifying unique sources of data and then providing a pathway for that information to be incorporated into your model. Not only will this data arrive in a huge number of formats, but it may also not always be available from one forecast to the next. Customers want reliability as well as uniqueness.
“The data can be a little wacky,” she says. “Sometimes it’s [something] buried in the deep mud of the Pacific that tells us just what the temperature was at a certain point in time. Sources can be wildly unstructured.”